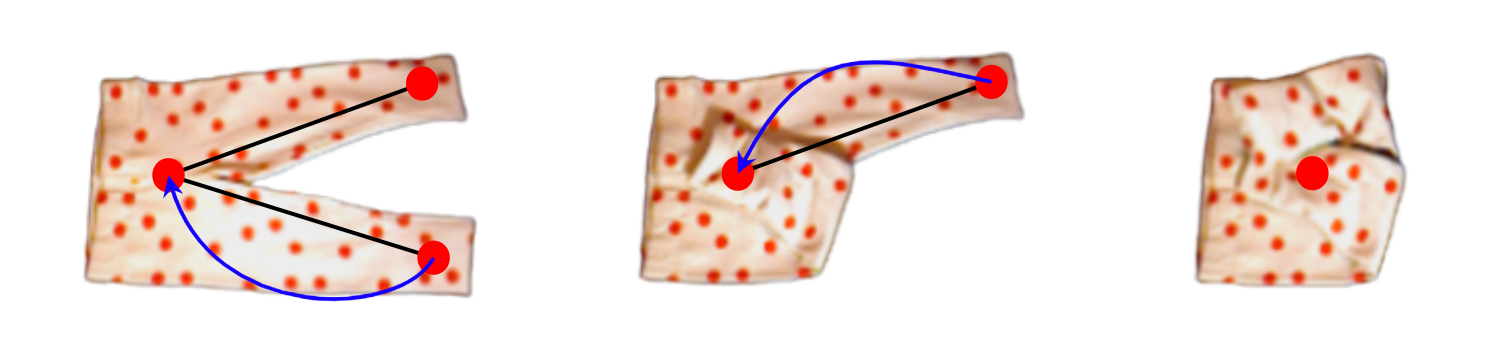
We study the problem of clothing item representation and build upon our recent work to perform a comparative study of the most commonly used representations of clothing items. We focus on visual and graph representations, both extracted from images. Visual representations follow the current trend of learning general representations rather than tailoring specific features relevant for the task. Our hypothesis is that graph representations may be more suitable for robotics tasks employing task and motion planning, while keeping the general properties and accuracy of visual representations. We rely on a subset of DeepFashion2 dataset and study performance of developed representations in an unsupervised, contrastive learning framework using a downstream classification task. We demonstrate the performance of graph representations in folding and flattening of different clothing items in a real robotic setup with a Baxter robot.
Download Paper